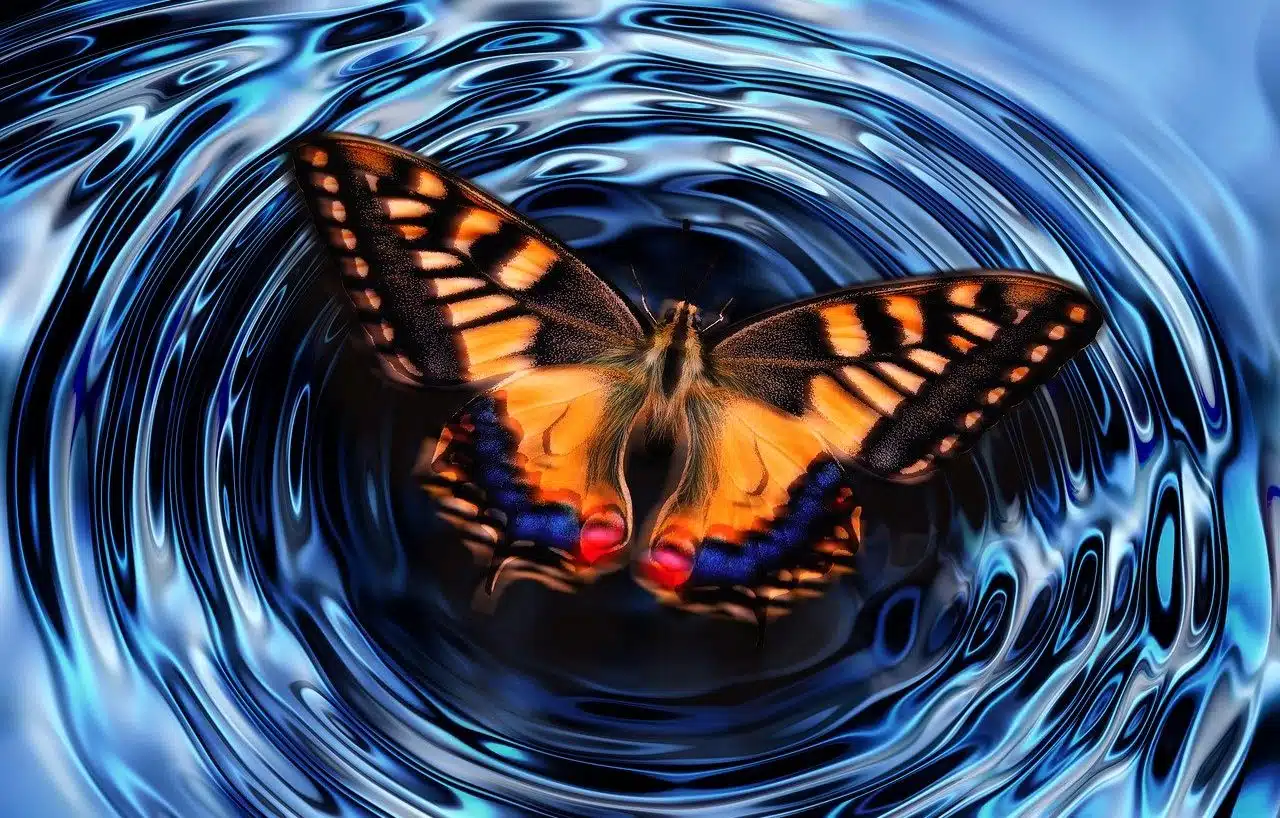
According to chaos theory, the evolution of a system is related to its sensitivity to initial conditions.
Chaos theory is a concept that is used in various sciences to refer to the changes that occur in a system when a variation in its initial conditions is recorded . These modifications can be very important even when the first alteration is minimal.
In deterministic systems, it is understood that behavior is determined by primary factors. This means that its development can be predicted. However, it is common for apparent randomness to break out at some point: this supposed randomness and instability are analyzed by chaos theory.
According to chaos theory , the future behavior of a system is associated with different variables. Due to this multiplicity of variables, the precision of the predictions cannot be guaranteed since a small variation in the original state is capable of causing numerous changes.
Origin of chaos theory
The origin of chaos theory is located at the end of the 19th century . The Frenchman Henri Poincaré is named as a pioneer in this area based on his research on the Solar System.
Poincaré , when studying the gravity of celestial bodies, noted the difficulties in determining the gravitational interaction that exists between three objects based on mathematical calculations. Therefore, with the three-body problem , he concluded that ensuring the stability of orbits is impossible. Analyzing these chaotic orbits, he arrived at the postulate that later became known as the Poincaré recurrence theorem , which states that some systems , after an extensive although finite time, return to a state close to or equal to the initial one.
Already in the second part of the 20th century , the American Edward Lorenz advanced chaos theory when he proposed creating a mathematical model to predict atmospheric conditions. Lorenz discovered that any difference in the starting point generated relevant modifications in the model predictions.
Thus, many scientists began to question the determinism of the laws postulated by classical physics. The branch of study that today is called chaos theory emphasizes the impossibility of predicting events in those dynamic systems that involve many variables. It must be taken into account that this chaos is present in nature but also in psychology, economics and other social sciences.
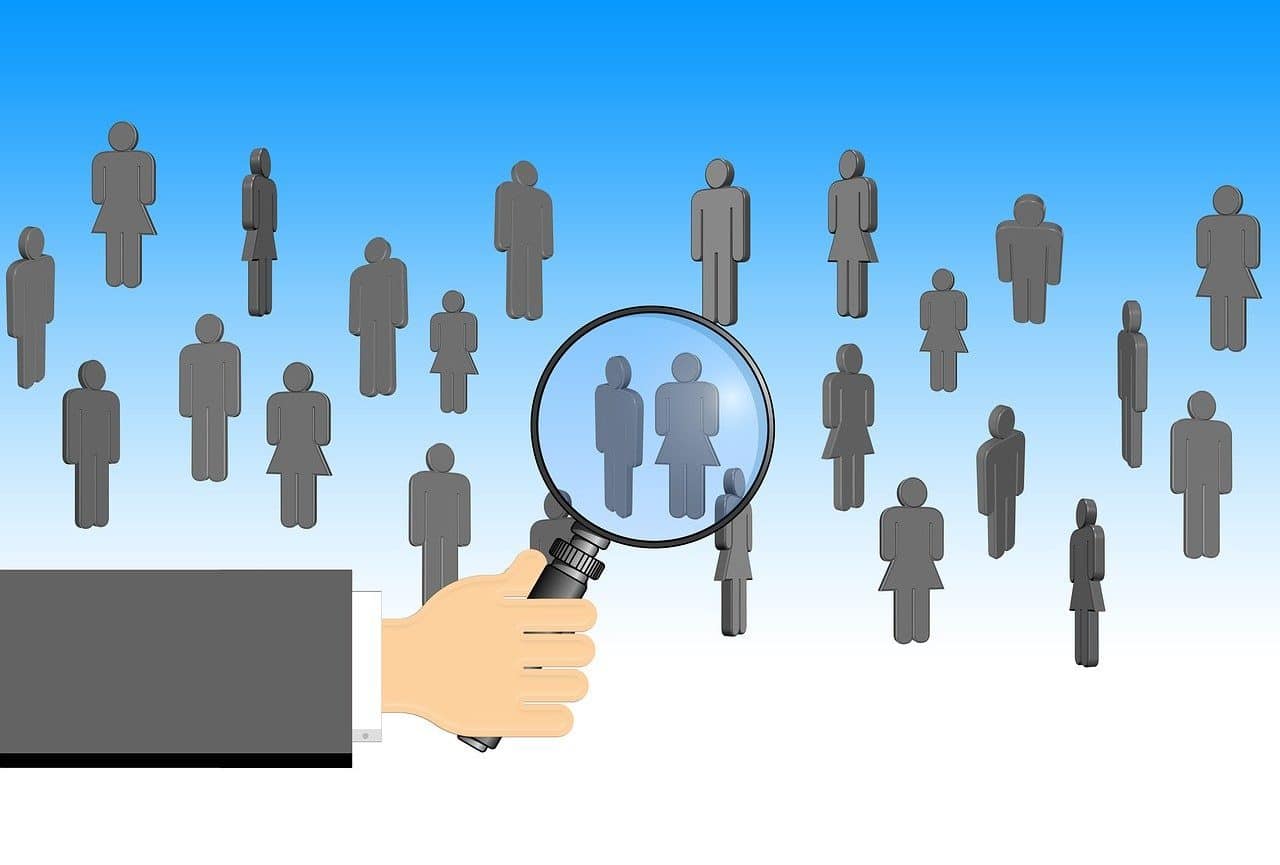
Climate models and population models can be studied from chaos theory.
The butterfly effect
Chaos theory is often associated with the so-called butterfly effect . This expression alludes to the fact that the simple flap of a butterfly's wings in one part of the world can cause a tsunami in the other end, according to an Eastern proverb.
What the butterfly effect indicates is that a seemingly minor event can produce a sequence of linked events whose results are unpredictable . The alteration in the initial conditions, therefore, generates a consequence that is impossible to anticipate in the first instance.
Chaos theory and the butterfly effect are part of dynamical systems. A dynamic system is made up of facts that change as time passes: when it shows a marked sensitivity to changes in initial conditions, it is classified as a chaotic system .
Although there is unpredictability in this chaos, its future creates patterns . That is why it is stated that chaos is not predictable but is deterministic, thereby ruling out chance.
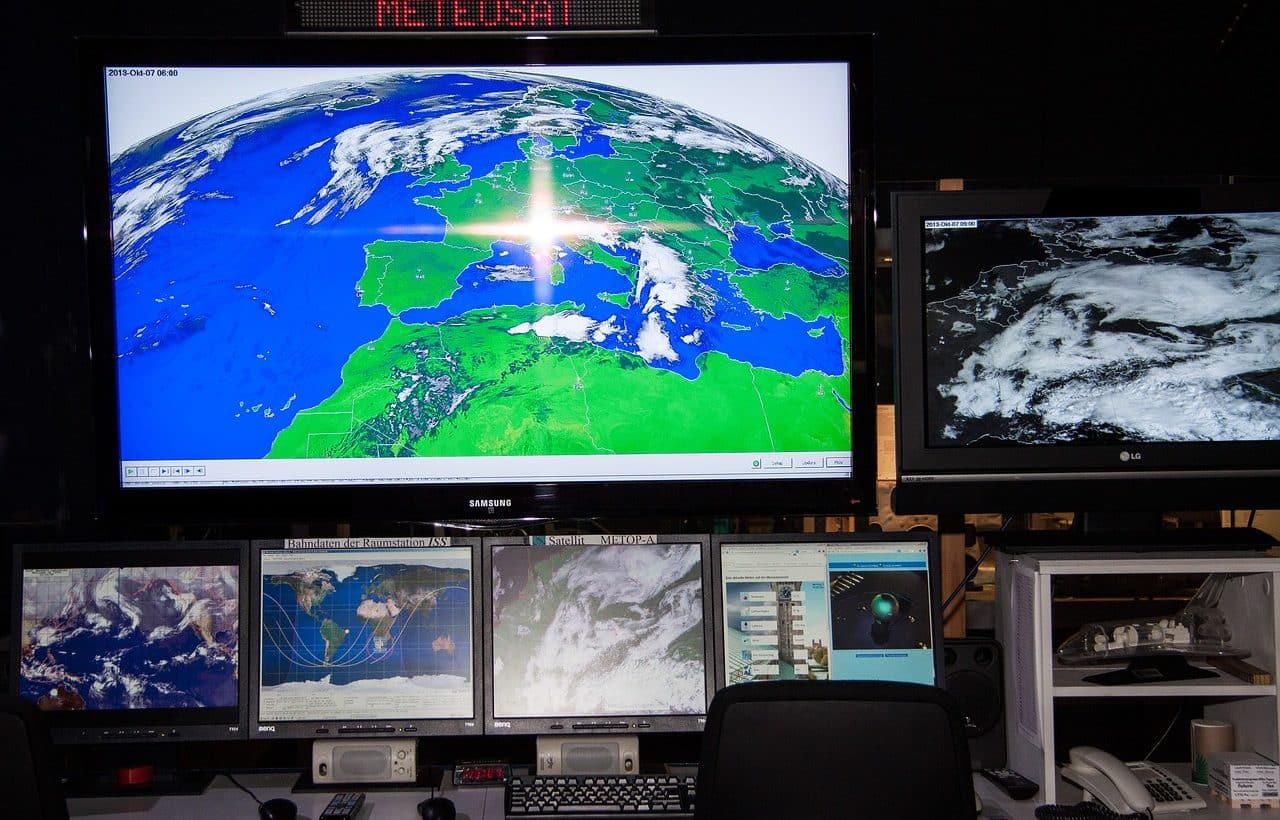
Climatology, meteorology and ecology are some of the sciences that are linked to chaos theory.
Attractors in chaos theory
It must be considered that even a chaotic system develops a trajectory with a tendency towards certain points. These points are called attractors .
Attractors are numerical values towards which, when evolving, a chaotic system tends. All those attractors of a system make up the set of fractals .
It can be said that a fractal is a mathematical object in which each of its parts resembles the complete object. This is because its structure is repeated at different scales.
It is possible to differentiate between classical attractors and strange attractors . In the case of classical attractors, all of their trajectories end in a stationary state. When this is not true and the sets cannot be described under the same parameters, they are strange attractors.
Neural networks and artificial intelligence
In the field of artificial intelligence, recurrent neural networks are used, which allow the introduction of linear orders.
It must be taken into account that, in a chaotic system, fluidity is present when there are changes in the connections between the elements due to different causes. An element that remains stationary can develop an activity spontaneously or through an interaction, generating the fluctuations in question. These fluctuations, when the system has a higher density of elements, tend to become periodic .
Returning to the question of artificial intelligence, information processing requires an internal order in the system so that data storage is possible. However, a degree of disorder is also needed for fluidity and, in this way, information can be processed.
This means that randomness must be introduced in artificial intelligence. By considering the properties of fractals, one can take a fractal section, discover its critical point, and study the data at the appropriate scale. Artificial neural networks, in short, suppose the convergence of an order and a disorder: input information has to pass through the network so that, after the execution of different operations, the output values are produced.