
The initial process in the construction of scatter diagrams and their descriptive interpretation.
A scatter plot is a type of graph used in statistics and data analysis to visually represent the relationship between two quantitative variables. Each point on the graph indicates a pair of values corresponding to the variables analyzed, which allows any pattern, trend or correlation between them to be observed. This type of diagram is especially useful in regression studies and in identifying linear or nonlinear relationships between variables.
Scatterplot Examples
Scatter diagrams are widely used to visualize relationships between variables in various disciplines. Some common examples include:
- Relationship between height and weight : In health studies, the scatter diagram is used to see how people's height and weight are related. This graph allows you to identify if there is a positive correlation , that is, if taller people tend to weigh more, or if the relationship is weak or non-existent;
- Sales and advertising trends : In marketing , a scatter diagram can show how advertising spending relates to the sales of a product. By representing data from previous campaigns, it is possible to see if an increase in budget is positively reflected in sales, helping to identify patterns and optimize future strategies;
- Temperature and energy consumption studies : In energy consumption analysis, the scatter diagram allows the relationship between outdoor temperature and energy consumption to be examined. This graph helps to observe if there is an increase in energy use during times of extreme temperatures (cold or hot) and to understand consumption patterns according to environmental conditions.
Scatterplot Types
There are different types of scatter diagrams that are used depending on the data patterns that you want to observe and analyze. Below are some of the most common ones.
Simple scatterplot
Each point represents a pair of values of two variables. It is useful to visualize if there is any type of relationship, whether positive, negative or non-existent, between the variables.
Clustered Scatterplot
The points are divided into different groups or categories using different colors or shapes. This approach allows you to analyze the relationships between variables based on a third categorical factor, such as gender, age or region, to obtain a more segmented perspective.
Scatterplot with trend line
It includes a trend line, usually calculated using linear regression, to highlight the general relationship between variables. The trend line helps to quickly identify the type of relationship, such as linear or curvilinear, and its direction.
Bubble diagram
Similar to a simple scatterplot , but with a third value that determines the size of each dot or bubble. This type allows three variables to be displayed simultaneously, being useful for analyzing data where a third variable influences the relationship between the first two, such as in market studies with sales, price and profit margin variables.

An approach to identifying patterns and correlations in complex data.
Descriptive and exploratory analysis
These methods are used to understand the structure and general characteristics of the data before applying more complex techniques:
- exploratory data analysis : involves preliminary exploring the data to identify patterns, outliers and underlying structures, using graphs and descriptive statistics;
- Categorical data analysis – Focused on data that belongs to categories or groups (such as gender, region, etc.), this analysis examines frequencies and relationships between categories.
Bivariate and multivariate analysis
When analyzing the relationship between two or more variables simultaneously, the following methods are applied:
- bivariate analysis : examines the relationship between two variables, as in the case of correlation analysis;
- Multivariate analysis : Also called multivariate data analysis , it involves multiple variables at once and helps discover complex patterns in high-dimensional data;
- analysis of variance (ANOVA) and multivariate analysis of variance (MANOVA) : determine whether there are significant differences between data groups. ANOVA examines one dependent variable, while MANOVA considers multiple dependent variables.
Predictive and regression analysis
These methods seek to identify trends and predict future behavior through mathematical models:
- regression analysis : evaluates the relationship between a dependent variable and one or more independent variables to make predictions;
- predictive analysis : uses statistical and machine learning models to anticipate future results based on historical patterns.
Component and factor analysis
These analyzes reduce data complexity and help identify underlying variables or common factors:
- principal component analysis (PCA) – reduces the dimensionality of data by transforming correlated variables into a smaller set of principal components;
- Factor analysis : Similar to PCA, it groups variables into common factors, explaining relationships between multiple observed variables.
Correlation and dependency analysis
To explore the relationship and dependency between variables, specialized techniques are used:
- Canonical correlation analysis : examines relationships between two sets of variables, identifying patterns of dependence;
- path analysis : studies causal relationships between variables, commonly represented by structural diagrams.
Complex data analysis
These methods are applied in studies where the data have special structures or present high variability :
- Cluster analysis – Groups elements into categories based on similarities, useful for market segmentation or pattern analysis in biology;
- spatial data analysis – examines data with geographic information to study the spatial distribution of phenomena;
- Longitudinal data analysis : studies data collected over time to observe changes in variables;
- mixed data analysis : combines quantitative and qualitative analysis to obtain a more comprehensive view of the phenomenon studied;
- Time series analysis – Analyzes sequential data to identify temporal patterns and make forecasts.
Survival analysis
This type of analysis evaluates the time until the occurrence of a particular event, being common in medical and reliability studies. Estimates the probability of an event occurring in a given time interval , useful in studies of mortality and product useful life.
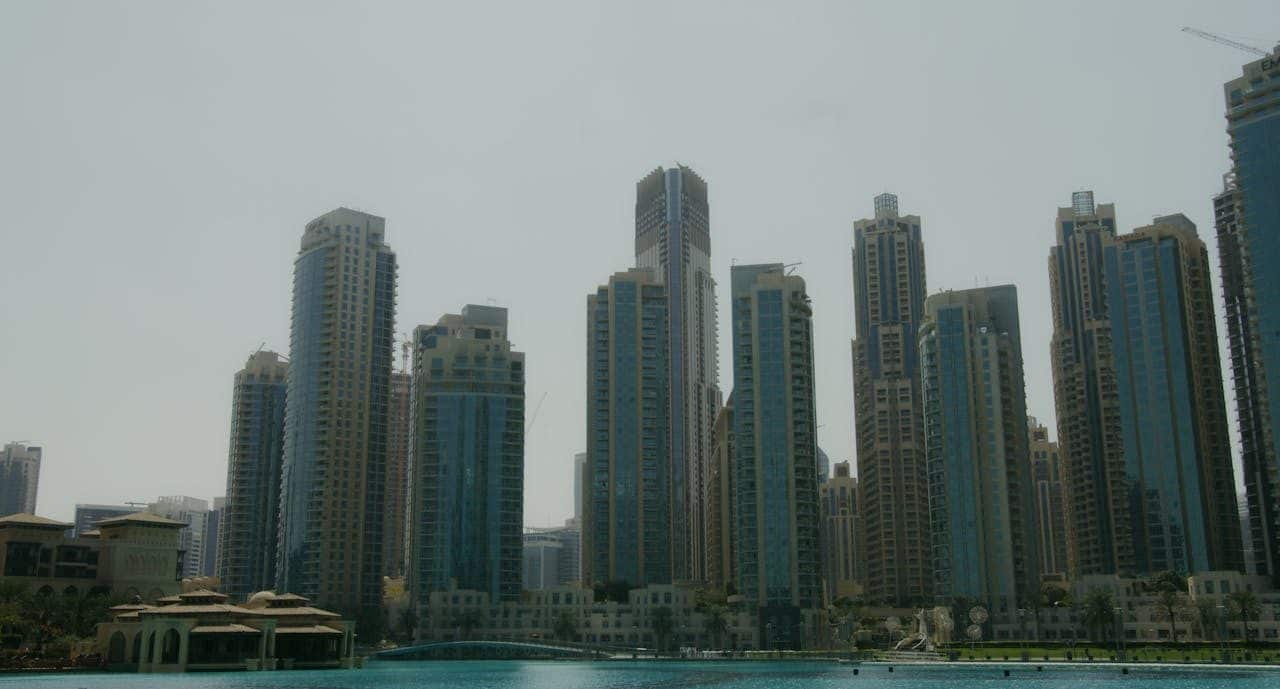
Classification of data into different categories or groups of importance.
Comparison with other types of charts
The scatterplot is just one of many graphical tools used to visualize and analyze data. Each type of chart has a specific purpose and is best suited for certain types of data and analysis objectives.
Below, some of the most commonly used graphs are compared along with the scatter plot to better understand their applications and differences.
Bar Charts and Pie Charts
They are categorical graphs that represent the distribution of data in groups or categories. The bar chart shows comparative values in vertical or horizontal bars, while the pie chart represents proportions of a total. Unlike the scatter diagram, they do not show relationships between quantitative variables, but instead illustrate distributions or percentages of categories.
Line Chart and Area Charts
Commonly used to represent changes over time, such as in time series analysis. Line charts are ideal for showing trends, while area charts emphasize the total volume below the curve. In contrast, the scatterplot focuses on individual points that represent relationships between pairs of values on the x-axis (independent variable) and the y-axis (dependent variable) , without needing to show temporal changes.
Control charts
Used in quality control, these charts monitor variations within a process to identify whether it is under control. Although both charts can include an X-axis and a Y-axis, the scatterplot seeks to identify relationships between variables, while control charts evaluate the stability of a process in a quality context.
radar charts
They are useful for comparing multiple categorical variables on a single graph, with each variable represented on a radial axis. This type of graph is suitable for comparative analysis of profiles, while the scatter plot is more used to observe correlations between two quantitative variables.
Charts in Excel
Excel is a widely used tool for creating statistical graphs, including all the types mentioned above. The scatter plot in Excel allows you to customize the X and Y axes to represent independent and dependent variables and add trend lines, making it an accessible tool for correlation analysis.