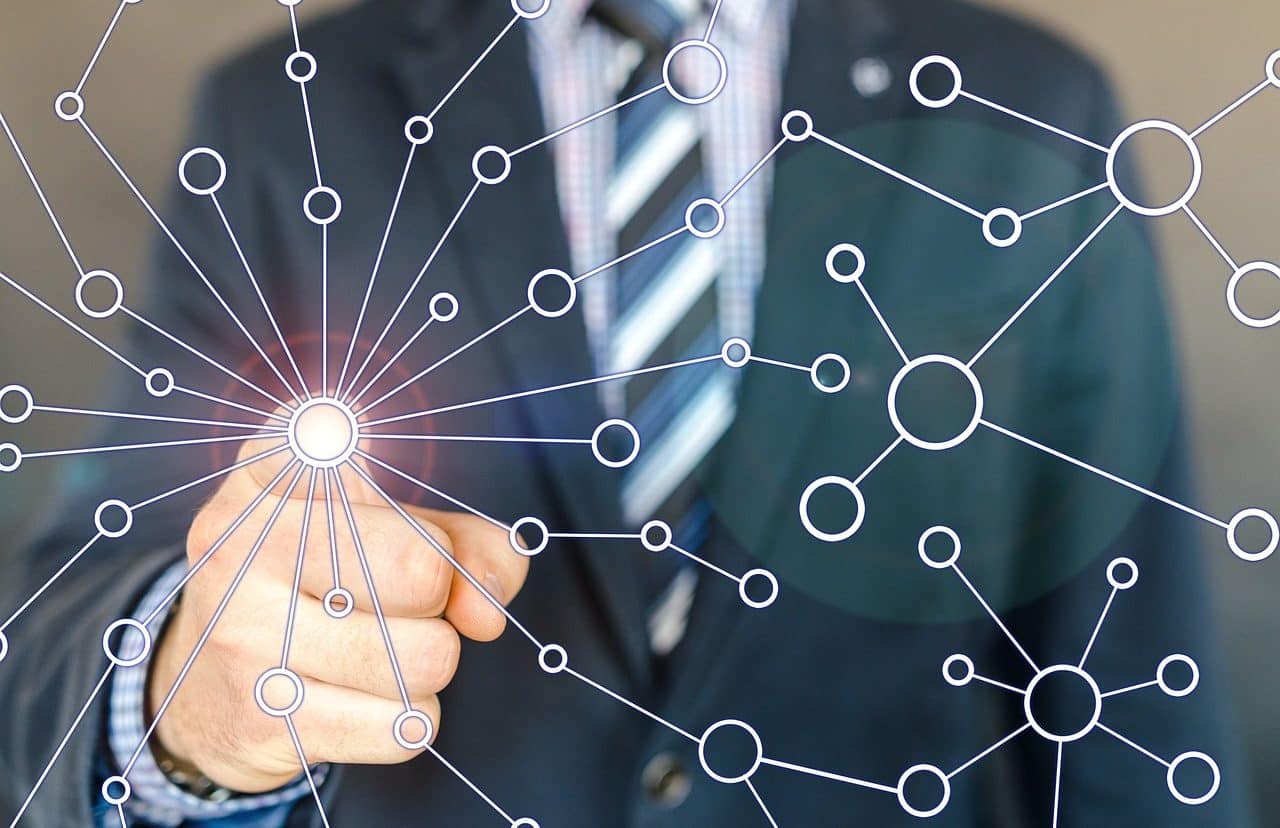
Factor analysis identifies underlying relationships between observed variables.
Factor analysis is a statistical technique used to identify underlying relationships between observed variables. The objective is to reduce their quantity to a smaller number of latent factors that represent underlying constructs, while maintaining as much original information as possible. This method is commonly used in social sciences, psychology, and marketing to simplify data complexity and detect patterns.
Examples of factor analysis
Below are some examples of how factor analysis is applied in different contexts.
Factor analysis in psychology
- Personality inventories : In personality assessment, psychologists use factor analysis to identify underlying factors such as extraversion, neuroticism, and openness to experience from responses to questionnaires;
- Intelligence tests : It is applied to determine the dimensions of intelligence, such as verbal reasoning, mathematical reasoning , and spatial abilities.
Factor analysis in marketing
- Market research : Companies use factor analysis to better understand consumer perceptions and attitudes toward their products, identifying factors such as perceived quality, customer satisfaction, and brand loyalty;
- Customer segmentation – Helps group customers into segments based on their purchasing behaviors and preferences, allowing for a more targeted marketing strategy.
Factor analysis in sociology
- Public opinion studies – used to analyze survey data and discover factors such as political attitudes, social values, and levels of well-being;
- Social behavior research : allows you to identify patterns in complex social behaviors, such as participation in community activities or media use.
Factor analysis in economics
- Analysis of economic indicators : used to identify the key factors that influence economic indicators such as GDP, inflation or unemployment;
- Financial risk models : In risk management, this technique is used to identify and quantify risk factors that can affect the performance of investment portfolios, such as interest rates, market volatility and credit risk.
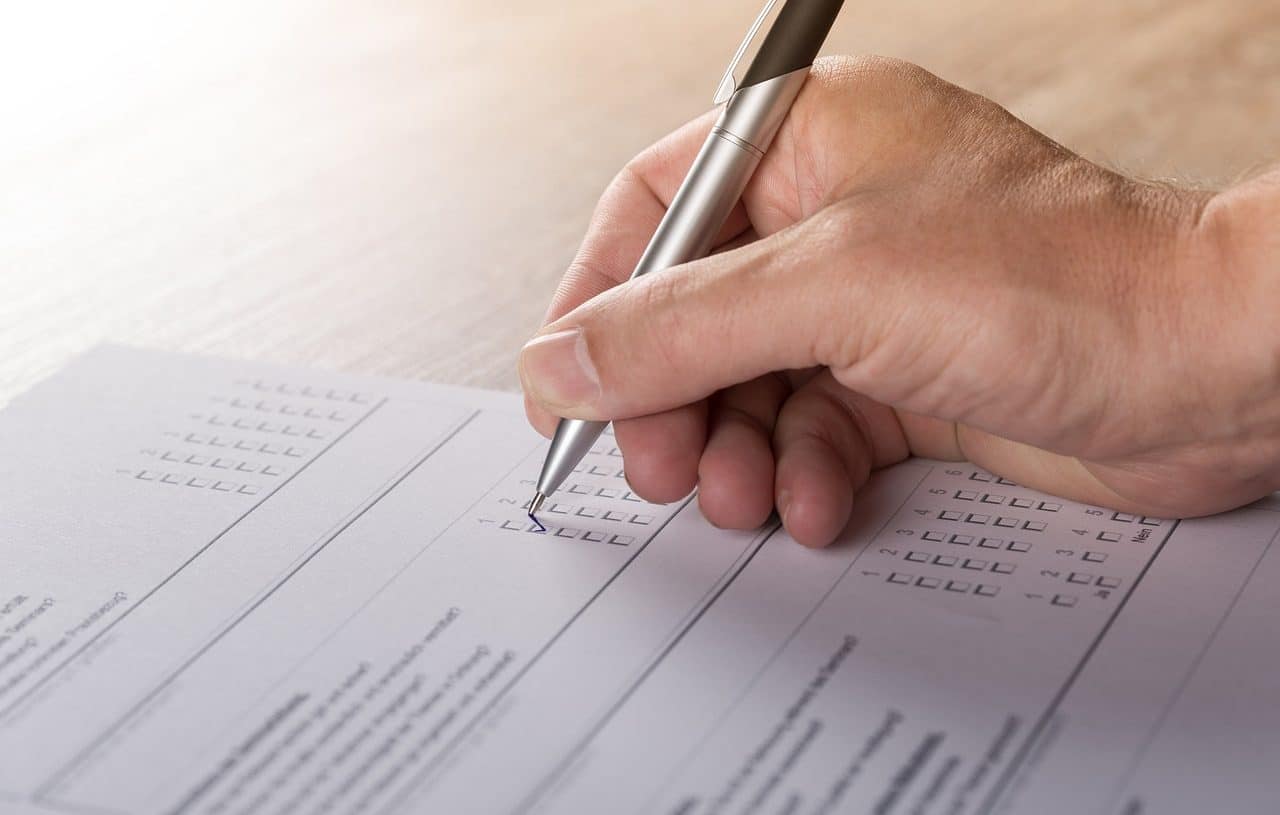
In psychology, factor analysis is seen in personality inventories and intelligence tests.
Latent variables
Latent variables are factors or constructs that are not directly observable, which are inferred from observable variables. In other words, they are underlying concepts that cannot be directly measured, but whose existence and influence can be deduced by analyzing patterns in observable data . For example, in psychology , intelligence or customer satisfaction are latent variables that are estimated through responses on questionnaires or tests.
Principal Component Analysis (PCA)
Principal components analysis and factor analysis are statistical techniques used to reduce the dimensionality of data, but they differ in their approach and objectives, since the former aims to transform a set of observable variables into a new set of uncorrelated variables called main components. These components capture the maximum possible explained variance of the original data, but are not necessarily related to underlying constructs. The PCA is purely descriptive and does not assume the existence of latent factors.
Exploratory and confirmatory analysis
Exploratory factor analysis (EFA)
It is used when there is no clear hypothesis or predefined model about the structure of the relationships between the variables. It is a technique that seeks to identify the quantity and nature of the latent factors that explain the correlations between observable variables.
Confirmatory factor analysis (CFA)
Confirmatory analysis is used when there is already a hypothesis or a clear theoretical model about the structure of the data. This approach is more rigorous and is used to test whether observable data fit a specific latent factor model.
Factor rotation
Factor rotation is a crucial step in factor analysis that is used to simplify and interpret the factors extracted during the analysis. After the underlying factors are identified, they are often rotated to achieve a clearer and more understandable structure. This redistributes the variance so that each factor is more clearly associated with a subset of variables.
Varimax
The most commonly used orthogonal rotation method. Its goal is to maximize the variance of the rotated factors, which simplifies interpretation by attempting to have each variable highly loading on a single factor and have low or near-zero loadings on the others. This makes it easier to identify clear patterns.
Orthomax
A family of orthogonal rotation methods that includes Varimax as a special case. Depending on a parameter, it can be tuned for different levels of simplicity or variance distribution. It is used when a more specific setting is needed.
Kaiser-Meyer-Olkin (KMO)
Index that measures the adequacy of the sample for factor analysis, evaluating whether the partial correlations between the variables are small. A high value (close to 1) indicates that factor analysis is appropriate. This index is calculated before performing the factor analysis to ensure that the data are suitable for factor analysis.
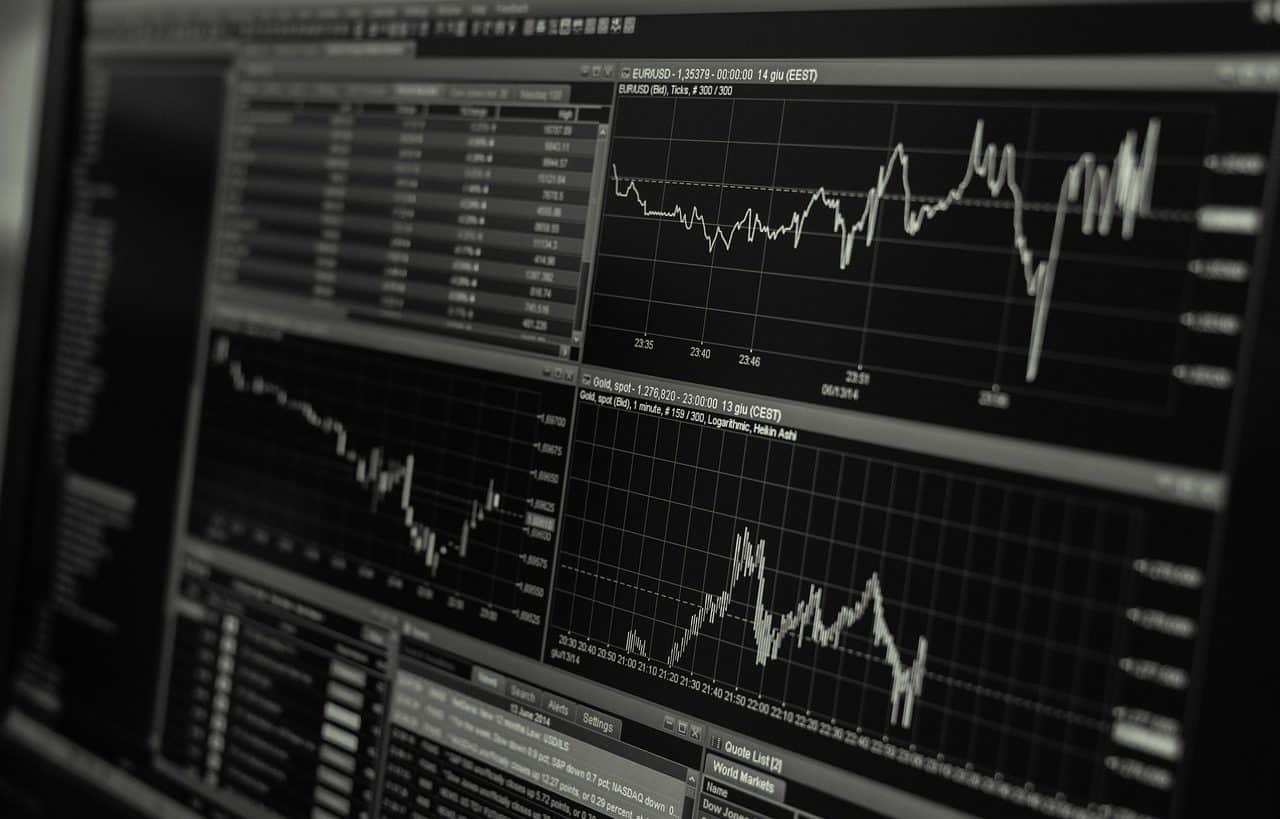
Analysis of economic indicators and financial risk models are two examples of factor analysis.
Data
The following concepts help determine how data should be treated in factor analysis and which techniques are most appropriate for each type of data.
Multivariate data
They include multiple variables measured simultaneously. In factor analysis, they are used to explore the relationships between different variables and identify latent factors that explain the correlations between them. Factor analysis allows us to reduce the dimensionality and reveal the underlying structure of these variables.
Categorical data
They are divided into discrete categories with no inherent order between them. Some examples are gender or type of product. In factor analysis, categorical data may require specific methods or transformations to be included, such as correspondence factor analysis.
Ordinal data
They have an order or hierarchy between categories, but the differences between them are not uniform or exactly quantifiable. A clear example is the satisfaction classification. In factor analysis, ordinal data are often analyzed using methods that can handle ordinal scales, such as factor analysis based on ordinal response models.
Continuous data
They can take any numerical value within a range and have significant, measurable differences. Height and weight are two common examples. In factor analysis, continuous data are commonly used and allow standard factor analysis techniques to be applied, such as principal components analysis (PCA) or exploratory factor analysis (EFA), since the variables are assumed to be normally distributed.
Methods
The following definitions provide a basic framework for understanding how these methods are applied in factor analysis, each with its own purpose and utility in different research contexts.
Extraction method
The technique used to identify latent factors from the observed variables. The most common methods include principal component analysis and common factor analysis. The choice of extraction method affects the identification and interpretation of factors.
Maximum likelihood method
Find the values of the latent factors that maximize the probability of observing the data. This method is useful when the data are normally distributed and allows for statistical tests of fit and comparisons between models.
Quantitative methods
Use of statistical and mathematical techniques to analyze numerical data and extract factors. They include procedures such as PCA, EFA and factor rotation, among others, with the aim of obtaining measurable and objective results.
Bootstrap methods
Sampling techniques used to estimate the precision of the estimates obtained in factor analysis. By generating multiple samples of the original data, they allow confidence intervals to be calculated for factor loadings and other statistics , improving the robustness and reliability of the results.
Mixed factorial methods
They combine different factorial approaches to analyze data that include both continuous data and categorical data. They can integrate classical factor analysis techniques with other statistical methods, allowing a more complete analysis when the data do not fit a single type of variable or when complex interactions between different types are desired to be explored.